By José Ignacio Orlando, PhD -Subject Matter Expert in AI/ML @ Arionkoder- and Nicolás Moreira -Head of Engineering @ Arionkoder-.
OpenAI, the very same company that launched DALL-E to generate images from text, has recently created a deep learning model that is able to transcribe voices in almost any language, regardless of how fast the person speaks or how much they mix in words from other languages. This revolutionary achievement in speech recognition is the consequence of leveraging massive amounts of data in combination with the newest AI models, in a vastly explored field at the intersection of signal processing and NLP.
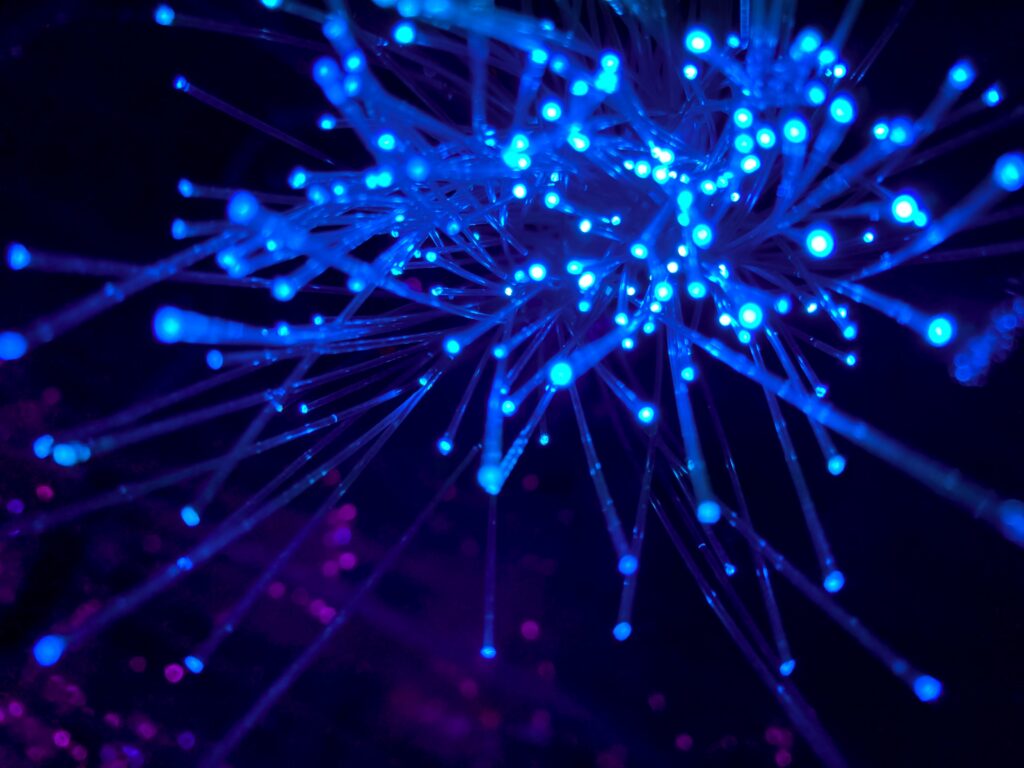
At the core of this model, named Whisper by its creators, is essentially a pretty standard deep neural network known as the Transformer, an architecture widely applied in NLP and, more recently, in Computer Vision. The novelty is then not in the method itself but in the way it was actually trained, using 680.000 hours of transcribed audio collected from the Internet. Yeah, more than 77 years of annotated sound, divided into pieces of 30 seconds and fed to a neural network for learning how to do the task. Neat, right?
This massive dataset was created by scraping several websites with transcribed voices from thousands of speakers in 99 different languages. While some of these pieces were manually transcribed by humans (such as subtitles in movies or YouTube videos), a big portion of them was automatically produced by other less accurate automated models. To avoid confusing Whisper with those samples, OpenAI crafted an automated method that discards them while keeping samples that use correct punctuation marks and capital letters. As a result, the dataset was clean enough to ensure a network that produces proper outputs, reproducing human ability to transcribe text and surpassing the accuracy of other much more complex and less usable architectures.
To further increase Whisper’s performance, Data Scientists made use of multitask learning, a machine learning discipline in which models are simultaneously trained to perform more than a single task at the same time. While this might sound counterintuitive, training models to do so allows them to leverage and discover common patterns between tasks, increasing their overall accuracy. In this case, OpenAI trained Whisper to simultaneously transcript audio to text, translate the output to English, recognize the input language, and identify if someone was speaking or not. This combined approach allowed the network to match or even outperform humans when doing speech recognition in multiple benchmark datasets.
Last but not least, OpenAI made a great contribution to the community by publicly and freely releasing both Whisper’s code and pretrained models. This paves the way towards a myriad of real-life applications, from aiding content producers to automatically subtitle their videos to allowing voice-guided assistants to be smarter when taking commands from humans. Furthermore, Whisper can be used to produce much better AI models, for example by collecting much larger corpuses of text by massively transcribing YouTube videos.
What other applications do you envision for speech recognition models like Whisper? Are you already considering leveraging this technology for your own business? Reach out to us at Arionkoder so we can help you achieve it!